Agenda
Predictive Analytics World for Healthcare Las Vegas 2018
June 3-7, 2018 – Caesars Palace, Las Vegas
This page shows the agenda for PAW Healthcare. Click here to view the full 7-track agenda for the five co-located conferences at Mega-PAW (PAW Business, PAW Financial, PAW Healthcare, PAW Manufacturing, and Deep Learning World).
Session Levels:
Blue circle sessions are for All Levels
Red triangle sessions are Expert/Practitioner Level
Workshops - Sunday, June 3rd, 2018
Full-day: 8:30am – 4:30pm
This one day workshop reviews major big data success stories that have transformed businesses and created new markets. Click workshop title above for the fully detailed description.
Two and a half hour evening workshop:
This 2.5 hour workshop launches your tenure as a user of R, the well-known open-source platform for data analysis. Click workshop title above for the fully detailed description.
Workshops - Monday, June 4th, 2018
Full-day: 8:30am – 4:30pm
This one-day session surveys standard and advanced methods for predictive modeling (aka machine learning). Click workshop title above for the fully detailed description.
Full-day: 8:30am – 4:30pm
Gain experience driving R for predictive modeling across real examples and data sets. Survey the pertinent modeling packages. Click workshop title above for the fully detailed description.
Full-day: 8:30am – 4:30pm
Dive in hands-on with crucial data prep steps, including cleaning, missing value imputation, feature creation/selection, and sampling. Click workshop title above for the fully detailed description.
Full-day: 8:30am – 4:30pm
This one-day introductory workshop dives deep. You will explore deep neural classification, LSTM time series analysis, convolutional image classification, advanced data clustering, bandit algorithms, and reinforcement learning. Click workshop title above for the fully detailed description.
Predictive Analytics World for Healthcare - Las Vegas - Day 1 - Tuesday, June 5th, 2018
Applied deep learning has fast become a standard tool for many industry machine learning applications. New advances in neural network techniques have opened the doors to solving problems at scale that were out of reach until recently. Because of these advances, applications such as in image recognition for self-driving cars, medical image classification, text translation, and fake news detection are both tractable and often the industry standard. In this keynote, Mike Tamir, who heads the data science teams at Uber ATG—the self-driving cars division—reveals how two key application areas of deep learning signal the broad importance of this emerging technology.
Data science, if judged as a separate science, exceeds its sisters in truth, breadth, and utility. DS finds truth better than any other science; the crisis in replicability of results in the sciences today is largely due to bad data analysis, performed by amateurs. As for breadth, a data scientist can contribute mightily to a new field with only minor cooperation from a domain expert, whereas the reverse is not so easy. And for utility, data science can fit empirical behavior to provide a useful model where good theory doesn’t yet exist. That is, it can predict “what” is likely even when “why” is beyond reach.
But only if we do it right! The most vital data scientist skill is recognizing analytic hazards. With that, we become indispensable.
DataRobot will demonstrate how innovations in Machine Learning & Artificial Intelligence can easily be leveraged to address a variety of opportunities and challenges across industries. Today, companies now have the ability to use machine learning & AI as a competitive advantage in their market, reduce operational costs, create new revenue streams, and drive higher customer satisfaction and loyalty. DataRobot's presentation will outline how scaling AI across your enterprise will deliver top & bottom line-benefits...create truly an "AI-Driven Enterprise".

Come join us to learn how one of UnityPoint's affiliates revamped their re-admissions reduction strategy using predictive modeling across the care continuum to reduce risk-adjusted re-admissions by 40% over three years. Under the hood are predictive models that forecast hospital length of stay, 30 day readmission risk, a heat map depicting likelihood of readmission each day post discharge, and follow up visit no show risk at the patient level each morning. We'll share the integrated tool, development details, and how use has spread organically across our health system.
Healthcare reporting and analytics has traditionally treated free text notes as a black hole. The Advanced Analytics team at OSF Healthcare has been leveraging natural language processing (NLP) to address this opportunity space for more than a year. Projects have included the extraction of Ejection Fractions from Echocardiograms, concept analysis of patient satisfaction surveys and named entity recognition for PHI and ailment identification. This session will provide a practical overview of NLP approaches the team has found useful and provide tips on how an organization can get started mining intelligence out of its free text without requiring large scale investments.
The need for timely decisions in ever-changing, unique scenarios is carved out in the healthcare field, where leveraging AI is expected to become a $6.6 Billion market in the next couple years. diwo combines the knowledge at hand with its Cognitive Framework to provide pre-packaged, quantified decisions, allowing knowledge to turn into the best possible action. A use-case demonstrating diwo’s cognitive decision-making for Readmission will be presented.
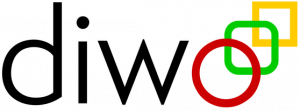
A modern health enterprise where business and clinical decisions are powered by advanced analytics stands in stark contrast to the existing status quo across health and life sciences today. Existing approaches to informatics — based in descriptive views of limited data sources — are incapable of supporting the sophisticated insights needed to optimize the tradeoffs between health outcomes and costs, and between standardized medical treatment plans and more personalized care practices. As discussed in the book Health Analytics: Gaining the Insights to Transform Health Care (Wiley, 2013), the health industry’s analytical lens must shift from the retrospective, presumptive, and population-oriented practices and policies commonly used today towards collaborative, data-driven, predictive, patient-centered, and real-time engagement-oriented processes. Insight-driven health requires integrated perspectives across health outcomes, financial management, risk management, performance management, and behavioral medicine. But the transition requires new business competencies, technical capabilities, and strong leadership.
Pharmaceutical industry operations are a complex network of interrelated business entities. There is an opportunity for fraud at each stage in the manufacturer/supplier-to-prescriber-to-dispenser-to-consumer distribution chain, and there are many known fraud schemes. We focused on the prescriber-dispenser-consumer end of the chain, and in particular, on schemes involving controlled prescription drugs.
Creating features from heuristics (domain experts) and deduction (graph analytics), which we employed in machine learning models, we developed a tool that can detect prescriber fraud schemes such as collusions, negligent prescribing and pill mills. Taya Fernandes, Drug Diversion & Data Mining Analyst, Medicaid Fraud Control Unit, Indiana, contributed to this presentation.
People with kidney failure treated by dialysis exhibit higher hospitalization rates than those without kidney disease, resulting in higher morbidity and mortality rates. Fresenius Medical Care developed, tested, and deployed a predictive model (with over 200 clinical and non-clinical variables) to identify patients at risk of hospitalization in the next year. Interdisciplinary clinical teams performed interventions with the identified high-risk patients. To date, we have observed a 23% reduction in average yearly hospital admission rates compared to controls in a regional assessment. This presentation will discuss the creation, implementation, and challenges with large scale deployment of this predictive model.
For children who have mental health conditions, starting therapies early is key for their future quality of life. Early and accurate screening tools are vital.
We present a clinically validated machine learning driven App to screen for autism. We give an overview of the development and optimization of two machine learning algorithms that use different media to identify autism, as well as the combination of their results, and the clinical validation of the algorithm's performance. We also present an algorithm to simultaneously screen for multiple conditions. We show its preliminary expected performance when outcomes of ADHD or autism are possible.

Predictive Analytics World for Healthcare - Las Vegas - Day 2 - Wednesday, June 6th, 2018
It is widely known that, while it delivers what is arguably the world’s best high technology care, the US healthcare system in general suffers from significant problems of equity, quality, safety and cost. Beginning in 2007 the Institute of Medicine called from a “Learning Healthcare System” which depends heavily on informatics. To provide that support requires that adoption of digital records which has now largely occurred; the ability of those record systems to share data which is the current challenge; and the analysis of that data to gain new medical knowledge that is fed back to the system as providers and patients make critical decision.
This talk will discuss the significance of and provide the basics of the new HL7 Fast Healthcare Interoperability Resource (FHIR) standard in both achieving interoperability and hosting practical approaches to providing analytic based tools to providers and patients. It will feature actual examples of tools developed using these new approaches.
Join DataRobot in this talk to learn how to apply Automated Machine Learning (AML) to help healthcare organizations drive ROI. We will discuss why AML is the future of ML/AI. We will share some common use cases that AML can help with and show a live product demo on a simple use case.

Successful care management (CM) programs begin with identifying the right people to manage. Identifying the wrong individuals can lead to a costly and inefficient allocation of limited CM resources. At CDPHP, we’ve developed a cloud-based framework for population health management using predictive models to identify members who would benefit from CM based on their prospective need for such services. Additionally, the models aid in triaging members to ensure they receive the resources best suited to their needs. Utilizing potential CM needs increases the opportunity to positively impact members’ health and limits false positives compared to traditional methods.
The ability of software to reason, answer questions and intelligently converse about clinical notes, patient stories or biomedical papers has risen dramatically in the past few years. This talk is intended for practicing data scientists and software engineers, and covers state of the art natural language processing, deep learning, and machine learning libraries in this space. We'll share notebooks & benchmarks from real projects using open-source software packages spaCy, Spark ML, TensorFlow, QuickUMLS, SyntaxNet and Spark NLP. The covered use cases will include clinical data abstraction, patient risk prediction, negation & temporal scope detection, topic modeling, and research paper classification.
The health care industry is seeing great advances in data-driven decision making, but lags behind several other industries that have considerably higher levels of adoption. Causes include the siloed nature of health care, the many professions involved, privacy rules, and long-standing hardened practices. Yet, if an organization does not develop a culture of analytics that permeates every level, department, and profession it will struggle to thrive with this powerful technology. This expert panel – moderated by Jeff Deal, who has managed teams in both worlds -- will explore important principles for sustained analytics success in health care.
MultiCare Health Systems’ OR administrators spent an inordinate amount of time sifting through a mountain of data to find insights about room utilization, number of cases, first case on-time starts, turnover, and other key performance indicators metrics. Building custom dashboards was labor-intensive and often didn’t contain all the information necessary for decision making. MultiCare found that having one source of truth that informs perioperative leaders and can “push” actionable insights is necessary to keep up with an ever-changing healthcare landscape. This session will detail how predictive analytics, machine learning, and cloud and mobile technologies helped MultiCare improve OR Operations.
The Low Carb Program is redefining chronic disease and wellness with over 275,000 global members and clinically-reviewed outcomes demonstrating 40% of people eliminate a diabetes medication and 1 in 4 completers reverse their type 2 diabetes at 1-year. The platform is global and engagement is completely AI-led, personalised to each patient and driven through real-time data feedback and insights.
Accurate data is necessary for purposes ranging from clinical decisions to service billing to scientific studies. However, with large volumes of data, error detection becomes difficult. To direct the efforts of coders poring through medical records looking for discrepancies in comorbidity reporting, the Data Science team at Fresenius Medical Care developed methods to detect patients likely to have unrecorded comorbidities. By using lab test results, natural language processing of rounding notes, and patient demographic information, we were able to improve the results of coders ten-fold. This session will discuss the development of these methods and the challenges along the way.
Workshops - Thursday, June 7th, 2018
Full-day: 8:30am – 4:30pm
This one-day session reveals the subtle mistakes analytics practitioners often make when facing a new challenge (the “deadly dozen”), and clearly explains the advanced methods seasoned experts use to avoid those pitfalls and build accurate and reliable models. Click workshop title above for the fully detailed description.
Full-day: 8:30am – 4:30pm
This workshop dives into the key ensemble approaches, including Bagging, Random Forests, and Stochastic Gradient Boosting. Click workshop title above for the fully detailed description.
Full-day: 8:30am – 4:30pm
This workshop demonstrates how to build uplift models (aka net lift models) that optimize the incremental impact of marketing campaigns, covering the pros and cons of various core analytical approaches. Click workshop title above for the fully detailed description.
Full-day: 8:30am – 4:30pm
Gain the power to extract signals from big data on your own, without relying on data engineers and Hadoop specialists. Click workshop title above for the fully detailed description.