Workshop sponsored by:
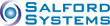
Workshop
Thursday, June 23, 2016 in Chicago
Room: Salon A4
Supercharging Prediction with
Ensemble Models
Intended Audience:
- Practitioners: Analysts who would like an introduction to ensembles or who would like to experience predictive analytics using a state-of-the-art data mining software tool.
- Technical Managers: Project leaders, and managers who are responsible for developing predictive analytics solutions, who want to understand ensembles, the approach that produce the most winning solutions in analytics competitions.
Knowledge Level: Familiar with the predictive modeling, especially decision trees.

Workshop Description
Once you know the basics of predictive analytics and have prepared data for modeling, how do you build models with the best possible accuracy? This workshop explains the principles of the most popular ensemble techniques found in software and used in analytics competitions: model ensembles. The instructor will explain Bagging, Boosting, Random Forests, and Stochastic Gradient Boosting. Attendees will build each type of ensemble using SPM, and adjust learning parameter settings to improve model accuracy.
Participant background
Participants are expected to know the principles of predictive analytics, especially decision trees. This hands-on workshop requires all participants to be involved actively in the model building process, and therefore must be prepared to work independently or in a small team throughout the day. The instructor will help participants understand the application of predictive analytics principles, and will help participants overcome software issues throughout the day.
Software
This workshop's hands-on experience is achieved using Salford Predictive Modeler software suite (SPM). A license will be made available to participants for use on that day (included with workshop registration). Ensembles can be built with many commercial and open source software packages, but SPM has particular features that make its ensembles unique in the industry.
Hardware:
Attendees will use their own laptops during the workshop. Please ensure that you have sufficient computing resources and permissions.
Recommended System Requirements
Because SPM is CPU intensive, the faster your CPU, the faster SPM will run. For optimal performance, we strongly recommend they run on a machine with a system configuration equal to, or greater than, the following:
- Pentium 4 processor running 2.0+ GHz.
- 2 GB of RAM.
- Hard disk with 40 MB of free space for program files, data file access utility, and sample data files.
- 2GB of additional hard disk space for virtual memory and temporary files (with the required space contingent on the size of the input data set).
- CD/DVD or USB drive to run the software installation
You must belong to the Administrator group to properly install and license. Once the application is installed and licensed, any member with read/write/modify permissions to the applications /bin and temp directories can execute and run the application.
Attendees receive a course materials book and an official certificate of completion at the conclusion of the workshop.
Schedule
- Software installation (if not already installed): 8:30am
- Workshop program starts at 9:00am
- Morning Coffee Break at 10:30 - 11:00am
- Lunch provided at 12:30 - 1:15pm
- Afternoon Coffee Break at 2:30 - 3:00pm
- End of the Workshop: 4:30pm
Instructor
Dean Abbott, President, Abbott Analytics, Co-Founder and Chief Data Scientist of SmarterHQ, Inc.
Mr. Abbott is an internationally recognized data mining and predictive analytics expert with over two decades experience applying advanced data mining algorithms, data preparation techniques, and data visualization methods to real-world problems, including fraud detection, risk modeling, text mining, personality assessment, response modeling, survey analysis, planned giving, and predictive toxicology.Mr. Abbott is the author of Applied Predictive Analytics (Wiley, 2014) and co-author of IBM SPSS Modeler Cookbook (Packt Publishing, 2013). He is a highly-regarded and popular speaker at Predictive Analytics and Data Mining conferences and meetups, and is on the Advisory Boards for the UC/Irvine Predictive Analytics Certificate as well as the UCSD Data Mining Certificate programs.
He has a B.S. in Mathematics of Computation from Rensselaer (1985) and a Master of Applied Mathematics from the University of Virginia (1987).