Tuesday, October 25, 2016
All sessions will take place in Room 1A10
8:00-8:30am • Room: Exhibit Area 1A
Registration & Networking Breakfast
8:30-8:35am
Conference Founder Remarks
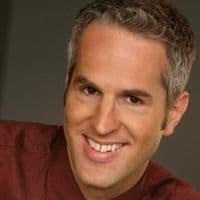
Founding Chair
Predictive Analytics World
Conference Chair Welcome
Keynote
Real World Data and the Transformation of Healthcare
The required transformation of healthcare will only occur if we understand the problems, create a practical strategy and understand the obstacles to transformation. Personalization is one of the critical strategic components. Traditional sources of population-based evidence have limited impact on personalization. Analyzing real-world data from large numbers of patients over an extended time period is essential for achieving personalization.
[ Top of this page ] [ Agenda overview ]
10:00-10:30am • Room: Exhibit Area 1A
Exhibits & Morning Coffee Break
Proactive Care Management
Case Study: St. Joseph Healthcare
Real-Time, Clinical Driven Predictive Analytics for Care Management
Hear clinicians at St. Joseph Healthcare discuss use of predictive analytics to support care management and reduce repeat ED and inpatient admissions. Powered by real-time clinical data from their statewide HIE, they identify patients at risk for serious illness, high cost, and repeat services. With this information, they engage patients early to improve outcomes and prevent serious events. Learn how they use analytic tools to target specific chronic diseases, identify care gaps, and allocate resources to those most in need. They'll also explain how these tools fit into their new care management model and help prepare for risk-based contracts.
[ Top of this page ] [ Agenda overview ]
Improving ER Utilization and Efficiency
The Best of Both Worlds: Predictive Modeling Using Both Health Plan and Hospital Data
Hospital readmissions are viewed as important indicators of the quality and efficiency of hospital care and are a significant component of our nation's healthcare costs. The Affordable Care Act authorized the Centers for Medicare and Medicaid Services (CMS) to lower reimbursement to hospitals with excess risk-standardized readmission rates for Medicare patients with certain conditions. Predicting which patients are at risk of readmission is an important problem that impacts both the payor and provider of hospital care. UPMC deploys both a payor-side readmission model built off the health plan's administrative claims as well as a separate provider-side, peer-reviewed model using inpatient clinical data. This talk will present the resultant hybrid model formed by combining the risk scores from both of these models.
[ Top of this page ] [ Agenda overview ]
12:05-1:40pm • Room: Exhibit Area 1A
Lunch in the Exhibit Hall
Lunch and Learn
Practical Advice for Integrating Predictive Analytics Into Your Clinical Care Management Workflow
Join clinical leadership from St. Joseph Healthcare for a conversation about their use of predictive analytics to support care management across a community health system. They'll detail the predictive tools and processes they're using to reduce readmissions, target chronic diseases, identify care gaps, prepare for risk-based contracts, and allocate resources to those most in need. See the software they're using up close, hear their impressive results, and learn how their success could be replicated at your hospital, health system, or ACO. They'll leave plenty of time for conversation, so bring your questions about predictive analytics, care management, value-based care, machine learning, and precision health.
1:40-2:30pm
Special Plenary Session
Doing Space-Age Analytics with Our Hunter-Gatherer Brains
Predictive Analytics is so powerful and so useful � everywhere � we are astonished that its widespread adoption has taken so long. Its modest risk and phenomenal return should lead rational actors to cooperatively pool technical and domain expertise to tweak production processes to the benefit of all. And yet, most early projects fail to be implemented � felled by fear, pride, and ignorance.
But we can anticipate those foes! Recall that success requires solving three serious challenges: 1) Convincing experts that their ways can be improved, 2) Discovering new breakthroughs, and 3) Getting front-line users to completely change the way they work. No wonder there is resistance at every stage!
John argues that it's helpful to have a mental model of the human brain as not optimized for success in our modern life of safety and abundance, but for survival within a small tribal society. And that with this model we can better anticipate � and escape - the traps that we idealistic techno-nerds tend to blunder into as we try to bring life-changing fire into the tribal circle.
Device Failure Analytics
Reducing Service Costs by Predicting Device Failures
Medical device failures can cause disruption to clinical staff, loss of productivity, and it affects the patient's health and their overall experience. Device errors also create extra service costs and can interrupt resources that are allocated for proactive maintenance. We combined devices that are predicted to fail with planned site visits to optimize proactive device maintenance. Discussions on lessons learned with deploying predictive models in a clinical environment , minimizing disruption and costs related to new predictive processes, and ROI tracking to ensure the deployed model is providing value to the organization.
Increasing Program Enrollment
Ready to Quit? Using Predictive Analytics to Increase Enrollment in Smoking Cessation Programs
Kaiser Permanente Southern California developed and implemented a predictive model to predict member readiness to enroll in smoking cessation programs. The model was deployed with 40,000 smokers across the entire region and has resulted in 5.6 times the expected enrollees as compared to random enrollment.
[ Top of this page ] [ Agenda overview ]
3:25-3:55pm • Room: Exhibit Area 1A
Exhibits & Afternoon Break
Early Warning System Development
Case Study: University of Virginia Health System
Developing a Custom Severe Sepsis Early Warning System for Deployment into Epic
Sepsis, the systemic response to infection, is the leading cause of in-hospital mortality. An improved early warning score (EWS) would enable earlier identification of patients with sepsis while reducing in-hospital mortality. We analyzed routinely available physiological and laboratory data to develop a new EWS for severe sepsis. This EWS was developed using cutting-edge Bayesian regression techniques, and then translated into rules capable of being deployed into the Epic Scoring System. In this presentation we will share the methods used to develop the model as well as the results of the model itself.
[ Top of this page ] [ Agenda overview ]
Retrospective and Real Time Clinical Evaluation
Predictive Models for Retrospective and Real Time Evaluation of Septic Shock
Early and accurate prediction of septic shock is critical to effective treatment/prevention of associated mortality and predictive models have shown great promise in this area. Retrospective identification of true cases allows for evaluation of interventions designed to improve outcomes and overcomes significant challenges associated with inaccurate diagnoses, and time consuming case review. We will discuss algorithms in development at Mercy for both of these applications as well as discuss possibilities for deployment of the real time algorithm in critical care settings, leveraging state of the art big data infrastructure.
5:30-7:00pm • Room: Exhibit Area 1A
Networking Reception
[ Top of this page ] [ Agenda overview ]
Day 2: Wednesday, October 26, 2016
All sessions will take place in Room 1A10
8:00-9:05am • Room: Exhibit Area 1A
Registration & Networking Breakfast
Conference Chair Welcome
Keynote
Predictive Analytics, Genomics, and Precision Medicine - Separating the Hype from the Reality
Precision medicine has the potential to completely alter the way we treat and care for patients by using genetic information to provide customized healthcare. Despite all the hype, our current health IT infrastructure does not support the use of the patient related genomic data that already exists. We are currently generating massive amounts of genetic data on patients that is largely ignored and never makes it into the medical record in a computable form. The implementation of precision medicine in the clinical realm presents many challenges and opportunities for informatics and predictive analytics.
Dr. Ken Yale presents one of the first, large-scale, replicable, population-wide studies applying advanced predictive analytics and genetic testing to more precisely target wellness interventions in an otherwise healthy population. In 2014, Dr. Yale's organization used advanced analytics algorithms to predict and target persons at-risk for a chronic condition, then used genetic tests to both further refine the predictions and customize interventions. This is one of the first examples of the practical application of predictive analytics and precision medicine on a population.
[ Top of this page ] [ Agenda overview ]
Managing EHR Systems
Case Study: Montefiore Medical Center
Re-Engineering Electronic Health Record Systems to Facilitate Clinical
Analytics in the Intensive Care Unit
Clinical decision making in the intensive care unit is especially prone to information overload because of the high intensity monitoring and the acuity and complexity of the critically ill patient. Software-based clinical analytics solutions can complement EMRs by sorting through big data so that actionable care decisions can be made at the bedside. This talk will describe efforts to re-design clunky, non-intuitive EHR systems to help physicians deliver safer, more efficient, and higher quality care in the intensive care unit.Real-world experiences from a nationally recognized academic medical center will be shared.
10:50-11:15am • Room: Exhibit Area 1A
Exhibits & Morning Coffee Break
Preventative Care Analytics
Case Study: Omada Health
What Healthcare Can Learn from Netflix: Building Personalization and Optimization into Preventative Care
Behavior change is hard. To date, even the most effective preventative behavioral interventions include little (or no) personalization.
The data science team at Omada is changing that. We'll discuss how we've built machine learning and experimentation directly into our product - leveraging vast amounts of behavioral data to create a system that continually improves in maximizing participant outcomes. From creating a culture of data-driven product development to specific analytical techniques used to design and deploy a precision behavioral intervention, in this talk we'll outline the path toward targeting the right individual, at the right time in the most effective way.
12:00-1:15pm • Room: Exhibit Area 1A
Lunch in the Exhibit Hall
Keynote
Brain-Machine Interface Technology: Separating Hope from Hype
A critical step in any brain-machine interface technology is recording brain activity and deciphering it to extract the underlying intention or perception. In the context of movement, every action we take begins and ends as some evolving pattern of neural activity, within distributed motor circuits in the brain, that control body motion via the spinal cord. When someone suffers from neurological impairment, such as spinal cord injury or stroke, the link between this neural circuit and the body is disrupted leading to debilitating movement deficits. Do we know enough about the workings of the motor system to intervene in such cases and develop mind-controlled assistive technology to restore movement capability in the paralyzed? Can a predictive analytics framework enable us to reliably extract movement commands right out of the brain? Are motor brain-machine interfaces currently feasible both from clinical and economic standpoints? This talk will examine the state-of-the-art of this provocative neurotechnology and provide insights into some of these questions.
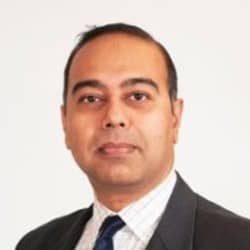
Assistant Professor and Director of Harvard
MIT Laboratory for Neuromotor Signal Processing
[ Top of this page ] [ Agenda overview ]
Sponsor Presentation
Big Data Challenges and Best Practices
Inexpensive technologies for collecting and storing big data have caused a paradigm shift: While analyses of healthcare-related data used to focus on statistical models and hypothesis testing, the vast amounts of new data (big data) now provide rich opportunities for discovery of novel and often unexpected patterns. Naturally, there are a number of challenges that must be addressed:
- First, commercial and open-source technologies for leveraging big data are rapidly evolving and often complex, requiring specialized resources to operationalize useful systems.
- Second, how can big-data systems and modeling approaches based on diverse technologies be standardized and documented as routine business processes? Predictive analytic models and modeling processes in healthcare must be repeatable, transparent, and validated.
- Third, how to make big data analytics methods widely available to business users such as physicians, healthcare workers, administrators, etc.
This brief presentation will discuss the relevant features in the latest releases of the Statistica analytics platform. Dr. Hill will provide overviews of system integration options, methods for in-database and in-memory (e.g., Spark) analytics, and model and analytic workflow management options for designing and publishing of web-interfaces (forms) for “citizen data scientists.”
Expert Panel
Predictive Analytics in Healthcare: What's Behind the Slow Adoption?
A recent survey of hospital executives indicated that only 31 percent of hospitals have been using predictive analytics for more than one year, and adoption among physicians is especially low. Up for debate is why predictive analytics is so slow to catch on within healthcare organizations and by practitioners when there is such a wealth of demonstrated value. Is it cost, lack of expertise, professional pride, or something else? Join our panel of healthcare experts as we consider this issue and explore ways to make predictive analytics more accessible to all healthcare providers at the institutional and individual level.
Panelists:
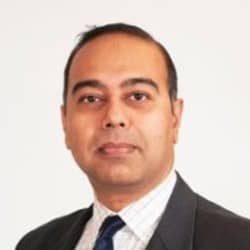
Assistant Professor and Director of Harvard
MIT Laboratory for Neuromotor Signal Processing
[ Top of this page ] [ Agenda overview ]
3:00-3:30pm • Room: Exhibit Area 1A
Exhibits & Afternoon Break
Clinical and Operational Decision Analytics
Predicting Colorectal Cancer Mortality
Having accurate, unbiased prognosis information can help patients and providers make better decisions about what course of treatment to take. Using a comprehensive dataset of all colorectal cancer patients in California, we generate predictive models that estimate short-term and medium-term survival probabilities for patients based on their clinical and demographic information. This talk will discuss how the model was developed, how it improves on previous models, how it should be used, and the impact on the approach to treatment of colorectal cancer patients.
Identifying Fraud
Case Study: Medicaid Fraud Control Unit, Indiana
Identifying Prescription Drug Fraud and Abuse
Over 46,000 people died from drug overdose in 2014, of which 28,000 stemmed from opioid abuse. The epidemic has garnered Federal and State attention.
One of the efforts to address the problem is the Prescription Drug Monitoring Programs (PDMPs), state databases housing controlled substances that were filled at pharmacies.
This presentation will demonstrate the power of applying advanced analytic methods to PDMP data for the detection of prescription fraud schemes. We will
show how we applied machine learning, geospatial filtering and graph analytics to identify individuals with drug-seeking behavior, doctor shoppers, as well as physicians and
pharmacies who aid and abet such activities.