Conference Day 1: Tuesday, October 25, 2016 |
8:00-8:45am • Room: Exhibit Area 1A
Registration & Networking Breakfast
Conference Chair Welcome Remarks
KEYNOTE
Weird Science: How to Know Your Predictive Discovery Is Not BS
"An orange used car is least likely to be a lemon." At least that's what was claimed by The Seattle Times, The Huffington Post, The New York Times, NPR, and The Wall Street Journal. However, this discovery has since been debunked as inconclusive. As data gets bigger, so does a common pitfall in the application of standard stats: Testing many predictors means taking many small risks of being fooled by randomness, adding up to one big risk. John Elder calls this issue vast search. In this keynote, PAW founder Eric Siegel will cover this issue and provide guidance on tapping data's potential without drawing false conclusions.
Diamond Sponsor Presentation
An Introduction to DataRobot Machine Learning Platform
Greg will introduce you to DataRobot, an automated Data Science platform, that makes building accurate models orders of magnitude faster and more deployable than any other technology available today. During this live demonstration, you'll see DataRobot in action and learn how it can be implemented in your business.
10:00-10:30am • Room: Exhibit Area 1A
Exhibits & Morning Coffee Break
[ Top of this page ] [ Agenda overview ]

Developing an Analytics Discipline in Recognizing Model Overstatement
In our Big Data world, reliance on machines and technology has become the governing philosophy particularly with advanced analytics involving predictive models. Technology has definitely enhanced the practitioner's capabilities but technology must be countered with the human perspective when developing predictive models. With an ever-increasing reliance on technology and machines, model overstatement becomes the more likely outcome. In this session, we explore the approach and tasks in identifying model overstatement. Through one case study with an insurance company, this above approach is used to not only identify model overstatement but why it has occurred.
10:30-11:15am • Room 1A25/1A26
Track 2: Online Marketing
Case Study: SmarterHQ
The Revolution in Retail Customer Intelligence
In this new era of Big Data, retailers collect data in ever-increasing volume and variety. In the midst of Big Data, a revolution is taking place in how retailers gain insights about customers, whether they interact with the brand online, in stores, or both. This session will describe the transition from reporting to data-driven decisions using predictive analytics. Success requires collecting the right data, creating informative derived attributes, making this data accessible in a timely manner, and building predictive models. Examples, drawn from real-world retailers, will include shopping cart funnel management, shopping cart abandonment, marketing attribution, churn, and purchase propensity.
[ Top of this page ] [ Agenda overview ]
10:30-11:15am • Room: 1A27

Case Studies: Telenor; US Bank
Uplift Modeling: Optimize for Influence and Persuade by the Numbers
Data driven decisions are meant to maximize impact - right? Well, the only way to optimize influence is to predict it. The analytical method to do this is called uplift modeling (aka, persuasion modeling). This is a completely different animal from standard predictive models, which predict customer behavior. Instead, uplift models predict the influence on an individual's behavior gained by choosing one treatment over another.
In this session, PAW founder Eric Siegel provides an introduction to this growing area to prepare newcomers for this PAW event's full-day track covering uplift modeling.
[ Top of this page ] [ Agenda overview ]
Track 1: Predictive Analytics for Sentiment
Case Study: A regional Bank in the Northeast US
Sentiment Unchained: Accelerating Analytics to Understand Customer
Behavior
The customer is always right; but what if they don't know what they want? Measuring satisfaction, sentiment or churn using traditional methods with limited data often belies true customer intent. But customers telegraph their next moves indirectly; and when actions speak louder than words, do you have the right tools to hear them? Building a predictive analytics workflow must include the ability to transform customer actions into features, tune an ensemble of machine learning algorithms, and interpret the model insights. But which actions, transformations and algorithms are effective?
This talk will focus on a recent data science engagement with a community-focused, regional bank in the Northeast. The discussion will focus on how to connect nebulous customer attitudes with actual profitability using machine learning to predict behaviors based on a small survey, then applied to all. MapR's Data Scientist Joseph Blue will review the results including how customer behavior has allowed this bank to apply these connections and identify untapped opportunities for growth.
11:20am-12:05pm • Room 1A25/1A26
Track 2: Economic Forecasting
Predicting Changes in Rental Prices in New York City Using Online Restaurant Reviews
The price of housing in major metropolitan areas in the US is affected by many variables including the economy, interest rates, the availability of jobs, and population growth. As an investor looking for higher yields from rental properties which neighborhoods should you invest in? In this work we introduce a novel data analytics approach to isolating the movement of different socio-economic groups through New York City. Using online restaurant reviews, we applied sentiment analysis, data clustering and data classification algorithms to provide a leading indicator for rental prices. Tawfik and Bari will cover these analytical results, which were produced by an extended group of graduate students and researchers at NYU.
Track 3: Uplift Modeling
Case Study: Miles & More (from Lufthansa)
Using Predictive Models for Demand Simulation - Purchase, Response and Uplift Modeling in Practice
The success of direct marketing campaigns is often measured by how many additional sales have been achieved. This measure is also known as uplift. By trying to predict the influence on an individual's behavior, uplift can be optimized. Recently, there have been a lot of analytical methods introduced to build uplift models. But is predicting influence in this way always the best option? Under certain conditions, standard predictive models provide even better results. Thomas Klein and Alexander Funkner share their experiences and present approaches to select the most suitable predictive model to maximize uplift.
[ Top of this page ] [ Agenda overview ]
12:05-1:30pm • Room: Exhibit Area 1A
Lunch in Exhibit Hall
KEYNOTE
Topic: Uplift Modeling
Persuasion Modeling in Presidential Campaigns and How It Applies to Business
Marketing, political campaigning, and healthcare have one major thing in common: organizations combine millions of individual interactions with social, digital, and traditional advertising in order to move people towards positive actions.
Prior to President Obama's reelection campaign, standard practices for persuading voters—that is, changing their minds—were unscientific and driven by long-standing assumptions and unsupported hunches. These practices mirror outreach efforts by other companies and organizations, who know that a certain percentage of their marketing efforts will inevitably be wasted on people who will not be the most receptive.
Daniel Porter of BlueLabs, who served as the Director of Statistical Modeling for the Obama Campaign, will discuss his experience using the results from a large-scale, randomized, controlled experiment that targeted persuadable voters for the Obama Campaign, i.e. persuasion modeling, aka uplift modeling. Daniel will review the ways these cutting edge statistical modeling techniques can be applied to influencing behavior in realms ranging from health outcomes to customer retention. He will also focus on methodological advances that have been made since 2012, how these techniques lead to more precise insights than ever, and how they can be used to drive broader strategic decisions in addition to narrower tactical choices.
[ Top of this page ] [ Agenda overview ]
2:15-2:35pm • Room 1A25/1A26
Gold Sponsor Presentations
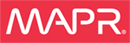
2:40-3:25pm • Room: 1A23

When Should We Trust Robots with Decisions?
The surge in big data and machine intelligence portends a future in which robots will have the capacity to transform daily life, as we know it. This raises a key question, namely, when should we entrust a decision to a robot? I provide a framework for business leaders for making this decision for any business problem. The analysis is based on two factors, namely, the inherent predictability of the problem and the consequences of errors.
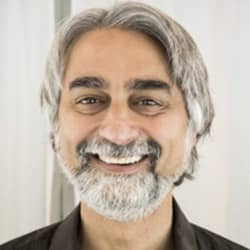
Professor, Stern School of Business & Center for Data Science, NYU
Editor-in-Chief, Big Data journal
2:40-3:25pm • Room 1A25/1A26
Track 2: Model Deployment
Five Best Practices for Deploying Analytic Models into Operations and Five Common Mistakes
We discuss five case studies to give an overview of some best practices for deploying analytic models into products, services and operational systems. We discuss commons architectures for deploying analytics. We then cover the role of vendor neutral standards for analytics, such as the Portable Format for Analytics (PFA) and the Predictive Model Markup Language (PMML). The case studies range from models built using R to models built using Hadoop, MapReduce and Spark. We conclude with five common mistakes that can delay the deployment of analytic models.
2:40-3:25pm • Room: 1A27

Causality in Business Analytics: Uplift Models and Directed Acyclic Graphs
Business people and policy makers typically want answers to causal questions, so that they may undertake actions that lead to results. The increased availability of data, along with developments in techniques of statistics and of machine learning, are making it easier to identify the causes of effects. For business, this has important implications, because it expands the ways in which "business analytics" can contribute to improved decision making. In this presentation, we examine two recent advances – uplift modeling (aka true lift modeling) and directed acyclic graphs (DAGs, aka Bayesian networks) – that are only now being applied to business decisions. We briefly motivate and summarize the techniques, and apply them to business examples. Our main conclusions are that these techniques are of practical value, that it is often possible to identify actionable causes, and that the challenge of transforming business analytics into causal business analytics remains relevant, even urgent.
3:05-3:25pm • Room: 1A23

Predicting and Managing Behavior In Process Industry Supply Chains
Process manufacturers create products including chemicals and tires. This industry is core to our economy and a single manufacturer can have tens of thousands of customers and vendors. Beyond the efficiency gains from automated supply chains is the predictive value that can be derived from the data. Automated decisions can impact operations days to months in the future and can save tens of thousands of dollars. Additionally predictive insights support longer-term decision making, and structural improvements to the system that can drive even more value. This session will present real-world challenges seen in achieving a predictive supply chain and present a demand-sensing solution that makes supply chain predictions and recommends action.
[ Top of this page ] [ Agenda overview ]
3:25-3:55pm • Room: Exhibit Area 1A
Exhibits & Afternoon Break
Special Plenary Session
Case Study: Barclays
The Rapidly Changing Big Data Landscape: Balancing the Power with the Dangers of Confusion
The combination of economic drivers to migrate to Big Data with the need to leverage semi-structured and unstructured Data, and the emergence of the Internet of Things (IOT) are driving a dramatic shift in the Enterprise Data landscape. The rapidly evolving open source stack has accelerated the changes to a point of confusion. Today's data analyst faces a bewildering environment of technologies and challenges involving semi-structured and unstructured data with access methodologies that have almost no relation to the past. This talk will cover:
- Why Big Data is different and what is driving the change.
- Issues and challenges in how to make the benefits of predictive analytics fit within the application environment.
- How real-time data streaming and embedded predictive analytics have become a requirements. - Three case studies to demonstrate the challenges and benefits of Big Data Analytics across different businesses from small to large.
[ Top of this page ] [ Agenda overview ]
4:45-5:05pm • Room: 1A23
Case Study: LexisNexis
Predicting the Fate of Legislative Bills and Finding Effective User Analytics for Business Decision Making
LexisNexis has developed an advanced legislative tracking service that uses predictive analytics to learn the probability of a bill's passage. The models forecast how likely a bill will move to the next stage of the legislative process based on about 20 features, including measurements of the social influence of the bill's sponsor, political parties of committee members, and the likely roadblocks within a committee and in the larger legislative process. This session will also describe work at LexisNexis that uses predictive analytics to deliver complex legal search results and develop advanced user analytics for business decision-making.
4:45-5:30pm • Room 1A25/1A26

Case Study: The MITRE Corporation
Applying Machine Learning Techniques to Improve Quality
Continuous process improvement remains an imperative across multiple sectors. Advances in algorithms, computing capacity, and machine learning tools make it possible to leverage historical quality attribute data to gain insights into what can be done to improve quality. This case study presents the current status of MITRE research applying machine learning techniques to software quality and project attributes to predict software project outcomes (success, cost, and schedule). Using multiple alternative predictive models will allow MITRE sponsors to check their software quality results and make better investment decisions. This analytics process can be applied to government and industry sectors.
4:45-5:30pm • Room: 1A27

Case Study: AIG
Parametric Uplift Regression Models
The session will present an old technique for new analytics methodology for Netlift/Uplift models. A number of nonparametric techniques have proven popular to estimate uplift models, such as tree-based and random forest based techniques. In contrast, we will apply a traditional regression-based approach: Recursive Bivariate Probit Models. One advantage of this approach is that we can then easily simultaneously calculate, for each individual, the probability of response when included in a campaign, and, the probability of response when not included in a campaign, and, taking the difference, prioritize our campaign on those with the greatest differential or incremental effect.
Track 1: Acquisition Funnel for Higher Education
Case Study: Becker College
Enhancing the Quality of Predictive Modeling on College Enrollment
Predictive modeling has gained popularity in studying college enrollment due to fierce competition in higher education. To make informed decisions and allocate limited resources to improve enrollment, predictive modeling has been applied to challenge and change the traditional recruitment process. This session is intended for two learning outcomes:
- Participants who are not familiar with predictive modeling will learn how to lay out a plan to collect and build a comprehensive data infrastructure and conduct predictive modeling.
- Participants who have run predictive modeling will learn how to critically examine the quality of their predictive analyses.
5:30-7:00pm • Room: Exhibit Area 1A
Networking Reception
[ Top of this page ] [ Agenda overview ]
Conference Day 2: Wednesday, October 26, 2016 |
8:00-9:05am • Room: Exhibit Area 1A
Registration & Networking Breakfast
Conference Chair Welcome
Special Plenary Session
Doing Space-Age Analytics with Our Hunter-Gatherer Brains
Predictive Analytics is so powerful and so useful – everywhere – we are astonished that its widespread adoption has taken so long. Its modest risk and phenomenal return should lead rational actors to cooperatively pool technical and domain expertise to tweak production processes to the benefit of all. And yet, most early projects fail to be implemented – felled by fear, pride, and ignorance.
But we can anticipate those foes! Recall that success requires solving three serious challenges: 1) Convincing experts that their ways can be improved, 2) Discovering new breakthroughs, and 3) Getting front-line users to completely change the way they work. No wonder there is resistance at every stage!
John argues that it's helpful to have a mental model of the human brain as not optimized for success in our modern life of safety and abundance, but for survival within a small tribal society. And that with this model we can better anticipate – and escape - the traps that we idealistic techno-nerds tend to blunder into as we try to bring life-changing fire into the tribal circle.
Track 1: Workforce Analytics
Case Study: Paychex
Predicting Employee Churn with Anonymity
In the age of Big Data, ethics is becoming increasingly important when predicting behavior responsibly. This is especially true when using predictive analytics to understand the actions of consumers or employees. Join us in reviewing a case study where we apply predictive modeling to increase employee retention, while protecting employees from the invasive big brother perception.
10:05-10:50am • Room 1A25/1A26

Q&A: Ask Karl and Dean Anything (about Best Practices)
Preeminent consultant, author and instructor Dean Abbott, along with Rexer Analytics president Karl Rexer, field questions from an audience of predictive analytics practitioners about their work, best practices, and other tips and pointers.
10:05-10:50am • Room: 1A27

Case Study: Visible World
What Shall I Watch Now? Ensuring the Right TV Ads Are in Front of the Right Audiences
New technology in the television industry is allowing advertisers to be much more targeted in their approach to buying and executing advertising campaigns. The technology itself however is only effective to the extent that television-viewing habits are well understood. Predictive methods on top of advanced TV viewership data have provided the ability to accurately predict viewership patterns for specific population segments, allowing advertisers to generate significantly more targeted views per dollar on TV than has ever been possible. This session will show how these predictive technologies are deployed to increase the effectiveness of TV advertising.
[ Top of this page ] [ Agenda overview ]
10:50am -11:15am • Room: Exhibit Area 1A
Exhibits & Morning Coffee Break
Track 1: Social Activism Engagement
Case Study: Change.org
Anatomy of a Social Movement: Using Analytics to Change the World
Change.org is the world's largest online platform for social activism. With the unique advantage of scale, our platform is continually fueled by analytics and data Science. In this talk, I'll discuss how algorithms such as collaborative filtering, graphs and topic classification are leveraged, as well as analytical techniques Change.org has developed and regularly use - that are unique to our mission. Using case studies from a variety of recent social movements, I'll discuss the role of data in key flows, including starting petitions, user growth, engagement, our client relationships, and the decision makers who are petitioned.
11:15am-12:00pm • Room 1A25/1A26
Track 2: Workforce Analytics
Predictive Job Maps: Optimizing a Workforce with a Network of Predictive Models
Where do employees' career paths flow within an organization? Can predictive intelligence inform and form this emergent order? There are at least three mappings of employee growth in organizations:
- Formal, usually hierarchical maps of expected progression, as planned by HR management
- Emergent, sometimes surprising pathways actually taken, which can be modeled with Markov Chains and Graph Theory, and
- Optimized pathways driven by KPI-focused predictive models, which may suggest novel and useful pathways for employee growth.
This discussion will explore these mapping methods, the interfaces between them, and directions for further innovation.
Track 3: Marketing Applications
Case Study: Bitly
Predictive Analytics for Different Business Types: Optimize All the Funnels
Understanding different types of customers' behaviors and developing data-driven business strategies accordingly can be one of the most valuable data science applications. By developing in-house predictive analytics tools, marketers will be able to gain a much deeper understanding of how to manage customer relationships with each business type and to optimize all the funnels. In this session, I will discuss the various in-house predictive analytics that I have implemented at Bitly, including marketing qualified lead scoring system, churn and upsell predictive models. Attendees will learn the techniques and machine learning models used, as well as key insights derived.
Track 3: Email Marketing
Case Study: Mountain America Credit Union
Maximizing Net New Deposits of a Promotional Email Campaign with Predictive Analytics
Leveraging data from past campaigns, Mountain America Credit Union was able to identify characteristics of the "ideal" responders (those more likely to bring "new new money"). The predictive model was leveraged to filter a refined list of customers for a future promotional campaign. Pilot results were compelling: test group had a response rate 14 times that of the control group; test group deposited 50 times more money than the control group; test group deposited new money at a 57 percentage point higher rate than the control group; deposits per account in the test group were 60 times the control group.
[ Top of this page ] [ Agenda overview ]
12:00-1:15pm • Room: Exhibit Area 1A
Lunch in Exhibit Hall
Keynote
Case Study: CA Technologies
The Impact of Analytics and Digital Transformation on Humans
The term digital transformation does not even come close to conveying the fundamental metamorphosis that is going on in the world. It is not just companies that are transforming. It is the human race that is transforming. Envision a world where robots are smarter than humans. They assimilate knowledge at a much faster pace compared to humans because they are connected to each other, and to all the devices through internet. Sounds like something out of a scary sci-fi movie? Be ready, it is going to be reality. Big data is playing a major part in this transformation.
Big data analytics technologies, algorithms, and concepts are the foundation of this digital revolution. These trends are disrupting business models. Who would have thought five years ago that the livelihood of taxi companies will be impacted by democratization of cabs by Uber!! Now, the financial industry is about to be disrupted by block-chain technology. Every business model disruption disrupts lives.
How does one survive in such a world? Quoting the Borg from the TV series Star Trek, "Resistance is futile!". Be part of the revolution. Be very adaptable. Scale up your own capabilities and keep transitioning into jobs that cannot be replaced by artificial intelligence and robots in your lifetime. Even the "maker" is no longer safe. Data Scientists have been the creators of analytics. They used to be, and still are a hot commodity in the market. But technologies are emerging that will commoditize this role in the next two years.
In this thought leadership keynote presentation, Saum Mathur will talk about the disruption that is coming, and how companies and employees should participate in it to stay relevant.
2:00-2:15pm • Room 1A25/1A26
Lightning Round of Vendor Presentations

Expert Panel
Data Prep: Overcoming the Bottleneck and Nailing It
Machine learning reigns supreme - but, alas, you first need examples from which to learn. The vast majority of hands-on time for most predictive analytics initiatives is spent preparing the data. Estimates generally hover around 80%. To realize the great potential of predictive modeling, you must form training data that consists of one row per training example, each consisting of a list of predictor (independent) variables, plus the target (dependent) variable that you plan to model/predict. The data prep is always a specialized task, which can only at best be partially automated and is replete with elusive pitfalls and unexpected delays. This expert panel will address:
- Tricks of the trade for data prep
- Tools, methods, and techniques
- Anecdotal successes and hard lessons learned
Panelists:
[ Top of this page ] [ Agenda overview ]
3:00-3:30pm • Room: Exhibit Area 1A
Exhibits & Afternoon Break
3:30-4:15pm • Room: 1A23
Track 1: Enterprise Platform
Case Study: Johnson & Johnson
Analytics at the Speed of Global Business: Delivering an Analytics Technology Platform
This session will focus on analytics technologies such as data management and visualization, data democratization, predictive analytics and real-time insights. The use case will demonstrate how a Global CPG Company uses a next generation technology platform to drive analytics and insights globally. This platform can meet the demands of a global, evolving data environment with its scale-out and lab/factory information capabilities, and support hybrid data scenarios as well. It connects both Advanced Analytics and Insights functions. Traditionally, analytics and BI applications have been implemented conforming to specs and design requirements, but have failed in user adoption because end-users are unable to use the applications. The user experience and usability is not something that can be added to an application after IT has created it. With our platform, we decided to drive the development from the user prospective right from the beginning - with a true understanding of the users, the goals they have, and the process they expect the analytics platform to facilitate.
3:30-3:50pm • Room 1A25/1A26
Track 2: New Data Sources
Case Study: The Hive (U.N. Refugee Agency lab)
The Data Set You Can No Longer Ignore: Consumer Engagement with Social Issues
As a standard practice, the private sector leverages data science and predictive analytics to understand customer's motivations. Yet, many brands are missing out on important cues from another set of data: social issues engagement. In this session, Rita will detail how brands can bridge this gap through partnerships with social enterprises or philanthropic foundations. By sharing data sets and understanding how people engage around social issues, brands and social enterprises together have the power to shed new light on existing databases. Companies then gain the opportunity to unlock behavioral triggers and engage new and existing audiences in more effective ways.
3:30-3:50pm • Room: 1A27
Track 3: Leads Scoring, B2B, Sales Funnel
Case Study: IBM
Integrating Analytics and Design Thinking Approaches for Sales Order Propensity Prediction
This case study explores propensity of an order to close for a highly configurable server line. These servers have a long manufacturing and testing lead time, often requiring sellers to predict which order to 'build-ahead' even before it is closed in order to guarantee prompt delivery. Conventionally this process relies on human experience to determine which order to pre-build, which often result in high inventory and high costs to take machines apart if it was not sold. This presentation discusses how we employ Design Thinking, together with Analytics approaches to enhance stakeholder's engagement in the development and the adoption phases of the predictive solution in the pre-build decision-making.
[ Top of this page ] [ Agenda overview ]
Track 2: Social Data for Predictive
Case Study: Cars.com
Predicting Consumer Review Engagement and Sentiment Using only Readily Available Social and Demographic Data
One of the most universal, and unfortunately often dreaded, consumer experiences is with the automotive dealership. The vast majority of these experiences are actually positive and dealers need to ensure that these positive experiences result in public, online dealership reviews. This case study addresses the issue of whether it is possible to create actionable analytics based on currently available social and demographic data to determine which consumers are likely to generate a review and therefore positively impact the dealer's online reputation. This predictive analysis would actually make it possible to counteract the impact of disproportionate negative voices in the consumer social network – helping automotive dealerships – and likely other online reputation dependent businesses – create a more balanced and informative market view.
3:55-4:15pm • Room: 1A27
Track 3: Labor Demand
Data-Driven Hiring of Data Scientists
Hiring -- even for data scientists -- is often not very data driven. At The Data Incubator, we run a fellowship to train and place data scientists in industry that receives over 2000 applications per session and is more selective than Harvard. To cope, we have to rely on robust analytics and machine-learning for our admissions process to ensure we are finding the best talent for our hiring partner companies. We'll explain why most traditional keyword-driven screening processes do not work for finding data science talent and how to both streamline and build an automated data-driven screening process that filters for skills and talent, not keywords and hype. Our results are based on thousands of aspiring data scientists who would like to be.
Track 1: Thought Leadership
Case Study: MIT Int Soc for Chief Data Officers
How $1B Companies are Scaling the Chief Data and Analytics Officer Function
Data is the fuel that powers analytics. However, an enterprise's misaligned focus on data can erode an organization's ability to create a business impact with analytics. The "Chief Data Officer" role is now receiving three times as many Google searches as "Chief Analytics Officer". As large enterprises increasingly view data as a strategic asset, what roles should analytics leader take in order to ensure success? In this session, Richard Wendell of the MIT International Society for Chief Data Officers discusses strategies for success at this critical intersection between data and analytics.
4:20-5:05pm • Room 1A25/1A26
Track 2: Consumer Experience Optimization
Case Study: Verizon
Best Practices Enhancing Contextual Experience with Predictive Analytics
This session covers Consumer Experience enhancement deployment that employs real-time predictive analytics. Field deployments have now evolved for multiple generations and associated best practices are emerging. How are predictive segmentation techniques used for the anticipation of demand and consumer intent? How is consumer experience enhancement predicted and attributed to channels and touch points? What is the business impact of predicting Consumer Context for experience enhancement and how do we measure it? What are best practice modeling techniques and their relative business impacts? Predictive analytics field deployments will include examples spanning the Automotive, Finance, Retail, Transportation, and Wellness industries.
4:20-5:05pm • Room: 1A27
Track 3: Product Recommendations - Advanced Methods
Graph Database for complex problems - Creating Personalized Food Item Recommendations at Restaurants
Choosing what to eat is an everyday part of life. Our brains are able to assess thousands of options quickly and nearly completely subconsciously. However, the process is far from simple; each person has their own preferences, each restaurant has their own specialties, and each dish its own ingredients. Graph Databases offers a natural way to codify these classes of objects and complex relationships to build a better model than what be accomplished through a traditional relational database.